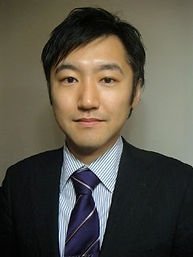
Producing a Diversity of Agent Using Repository-based Multi-Agent Framework
Keynote Speech 1 (Morning 28/11/2022)
Prof Takahiro Uchiya, Nagoya Institute of Technology
An agent is software that operates autonomously through interaction with people, the external environment and other agents. Typical properties of agents include autonomy, sociality, learning, mobility and promptness. An agent system is a system in which agents co-operate with each other and aim to solve problems by organising themselves with multiple agents. Since individual agents have only simple functions, the problem is divided into simple subproblems, which are then assigned to each agent. The solved partial solutions are then integrated to solve a single problem as an agent system as a whole. An agent framework is a framework for the operating environment that realises the properties of an agent. It provides communication protocols for sociality and reasoning mechanisms for autonomy. Currently developed agent frameworks include DASH, JADE, OMAS and SAGE, among which the DASH framework is the most capable of developing and operating agents with diverse agent properties. DASH (Distributed Agent System based on Hybrid architecture) consists of a repository, which is a server that collects and manages agents, and a workplace, which is the environment in which the agents actually run. DASH is called a repository-based agent framework because the system is developed based on a repository. By managing a wide variety of agents in a repository, a multi-agent system with various features can be dynamically composed and provided to users. This talk introduces the properties of agents that can be realised using the repository-based multi-agent framework DASH, and describes a case study of the development of an applied multi-agent system.
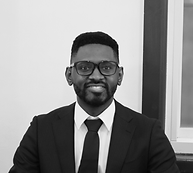
Connecting lifelong learning on the blockchain: key factors and lessons learned
Keynote Speech 2 (Afternoon 28/11/2022)
Dr Patrick Ocheja, Kyoto University
The advent of blockchain technology presents new opportunities to build multi-stakeholder systems in a truly democratic way. A typical use case is in education where the blockchain can be used to enable learners to connect and take their data with them across different learning platforms and schools. The importance of such learning data control and mobility includes privacy, reducing cold-start, improving personalization, ensuring learning continuity, and empowering learners to make smart data-informed decisions. In this talk, we present a novel blockchain of learning logs (BOLL) system that enables connected lifelong learning. Key factors from both the blockchain and education research that informed the design of the BOLL system will be presented. Finally, we share lessons learned that could be beneficial in building future decentralized applications on the blockchain.
Patrick Ocheja is a JSPS Research Fellow at the Academic Centre for Computing and Media Studies, Kyoto University, Japan. He recently received a Ph.D. in Informatics from the Graduate School of Informatics, Kyoto University. His current research focuses on the use of decentralized technologies in education and learning analytics. Dr. Ocheja also serves as a peer reviewer for many international journals and conference papers including those published by IEEE, ACM, Springer, and Taylor & Francis. Patrick has also won multiple awards including the IPSJ Yamashita Memorial award, best paper awards, and a best graduating student award.
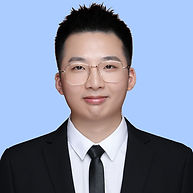
Influence-aware Incentive Allocation Problem under Uncertainty
Keynote Speech 3 (Morning 29/11/2022)
Dr. Shiqing Wu, University of Technology Sydney, Australia
In recent years, many applications have deployed incentive mechanisms to promote users’ attention and engagement. Most incentive mechanisms determine specific incentive values based on users’ attributes (e.g., preferences), while such information is unavailable in many real-world applications. Meanwhile, due to budget restrictions, realizing successful incentivization for all users can be challenging to complete. In this light, leveraging social influence to maximize the incentivization result is considered by many researchers. The incentive provider can directly incentivize influential users to affect more users, so the cost of incentivizing these users can be decreased. However, identifying influential users in a social network requires complete information about influence strength among users, which is impractical to acquire in real-world situations. In this talk, I will introduce some topics related to influence-aware incentive allocation problems, including the influence maximization problem, the traditional incentive allocation problem, and some related works. Meanwhile, our recent work on this topic will be introduced as well.
Shiqing Wu is currently a Postdoctoral Research Associate in the Data Science and Machine Intelligence (DSMI) Lab, Data Science Institute, School of Computer Science, at the University of Technology Sydney (UTS). He received his PhD degree in Computer Science from the University of Tasmania in 2022, and a joint B.Sc. degree in Computer Science from Auckland University of Technology (New Zealand) and China Jiliang University (China) in 2016. Shiqing has published several research papers in the journals and conferences related to AI, including IJCAI, AAMAS, WISE, PRICAI, Knowledge-based systems, etc. His research interests involve artificial intelligence, incentive allocation problem, influence maximization problem, social influence analysis, agent-based modelling, and machine learning.
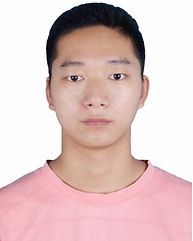
Multi-agent Modelling for Flight Reactionary Delay Prediction
Keynote Speech 4 (Afternoon 29/11/2022)
Dr. Qing Cai, Nanyang Technological University, Singapore
Flight schedules are highly sensitive to delays, and these delays can magnify and cascade as the flight itineraries progress, causing reactionary delays, due to the interconnected and interdependent nature of air transportation systems. An expeditious and green aviation can be warranted if the flight reactionary delay propagation dynamics can be traced in an effective way. In this talk, a multi-agent modelling approach for flight reactionary delay prediction will be discussed, given the magnitude of primary delays witnessed by the flights at the beginning of the itineraries. Every flight is modelled as an agent that functions in a dynamic air traffic system environment, receives information about other agents, and updates its own arrival and departure schedule. A case study on flights in Southeast Asia covering eleven countries is carried out to demonstrate the effectiveness of the proposed multi-agent-based approach.
Qing Cai got his PhD in Pattern Recognition & Intelligent Systems from Xidian University in 2015 under the supervision of Prof Maoguo Gong. After that, he worked as Research Fellow in collaboration with Prof Jiming Liu, Prof Mahardhika Pratama, and Prof Sameer Alam. Currently, he is a Senior Research Fellow at the Air Traffic Management Research Institute with Nanyang Technological University, Singapore. His research interests are complex networks, metaheuristic optimization, and deep learning, with applications to air traffic management.